A rolling statistical model making use of all YouGov data shows support for leaving the European Union increased in early June only to peak on June 13th
There has been a lot of noise in polling on the upcoming EU referendum. Unlike the polls before the 2015 General Election, which were in almost perfect agreement (though, of course, not particularly close to the actual outcome), this time the polls are in serious disagreement. Telephone polls have generally shown more support for remain than online polls. Polls from different polling organisations (and sometimes even from the same organisation) have given widely varying estimates of support for Brexit. The polls do not even seem to agree on whether support for Brexit is increasing or decreasing.
This lack of agreement partly reflects the fact that polling a referendum is more difficult than a general election, because general elections occur regularly and many patterns can be expected to stay the same from election to election, enabling us to learn from past mistakes. Referendums address unique questions that may turn out different voters, and may scramble the political loyalties that tend to persist from general election to general election. Still, as the Scottish independence referendum showed, it is possible to get pretty close to the right answer with careful polling and analysis. In this article, we describe a strategy we are using to synthesise the evidence from the many polls that YouGov runs, the resulting estimates of the overall referendum results and how it breaks down by geography and some other variables - as well as what might still go wrong with our approach.
OUR STRATEGY
The approach we are following, which is referred to as multilevel regression and post-stratification (MRP), has three components. Here we will use a bit of shorthand that is useful for describing the approach: when we refer to a ‘voter type’, we mean someone's measurable characteristics. This includes age, gender, educational qualifications, who they voted for at the last general election, where they live, and so on. So one type of (potential) voter in the referendum might be a female, 31-35 year-old, with a university degree, who voted Labour and lives in the Enfield Southgate constituency in London. For each of these types, there are three important quantities that we would like to know.
- What proportion of people of that type will vote Leave versus Remain, among those who do vote?
- What proportion of the individuals in each voter type will turn out to vote?
- How many (voting eligible) individuals are there of that type?
If we knew all three of these for every type, we could simply multiply the Leave and Remain vote shares by the turnout share by the number of voters of each type, add the results for every type together, and we would have the election outcome. The difficulty is in forming high quality estimates of each of these from the available sources of data that we have to work with.
What proportion of people of that type will vote Leave versus Remain, among those who do vote?
We estimate the proportion of people of each type who will vote Leave, among those who vote, using the YouGov panelists who have expressed an opinion on the referendum in the last two weeks. We estimate how support for Leave vs Remain varies as a function of 2015 general election vote choice, age, qualifications, gender and date of interview, as well as interactions of some of these. In addition to the ways that voters vary in their intentions by their individual characteristics, we also model how they vary on average by the constituency they live in.
What proportion of the individuals in each voter type will turn out to vote?
We estimate the proportion of people of each type who will turnout using a similar model based on individual-level and constituency-level variables, however, this model is not fit on the YouGov panel. We use the 2015 British Election Study post-election face-to-face survey, which validated turnout from the electoral rolls in addition to asking whether respondents voted. We use the BES to calibrate the likely demographic profile of turnout, rather than self-reported intention to turnout in this election, for two reasons. First, self-reported intention to turnout is well-known to be problematic. Second, and more importantly, we know that the YouGov panelists are more engaged than the average citizen. This means that, even if they all accurately predict whether they would turn out (which they probably do not), we would likely end up with the wrong demographic profile of voters overall. We have made a judgment call that we would rather use a high quality estimate of the patterns of turnout from the 2015 general election than a low quality estimate of the patterns of turnout for the referendum based on the historical stability of turnout patterns, but this is a key place where we might get things wrong if there is a large change in turnout patterns.
How many (voting eligible) individuals are there of that type?
We estimate how many people there are of each type in the electorate primarily based on the 5% microdata sample from the 2011 UK Census, with updated distributions of age, gender and educational qualifications from the 2015 Annual Population Survey. This data has local authority rather than parliamentary constituency, so we use the overlapping geography to impute each of the 3m records to a parliamentary constituency as well as a local authority. We then augment this data by imputing the general election votes of each of these 3m individuals using BES and YouGov survey data from around the general election plus the knowledge of exactly how many people voted for each party in each constituency. The logic of this approach is that, at the very least, we know we have the right number of 2015 General Election supporters of each party (and non-voters) in each parliamentary constituency, as well as the right mixes of age, gender, etc. If we err, it will not because of the same problems that led to the 2015 general election polling miss. In constructing our UK estimates, we also construct estimates for Northern Ireland as a whole based on other public polling and the size of the electorate there in 2015, and add in a rough estimate of the relatively small number of overseas voters (including Gibraltar).
RESULTS
Our current headline estimate of the result of the referendum is that Leave will win 51 per cent of the vote. This is close enough that we cannot be very confident of the election result: the model puts a 95% chance of a result between 48 and 53, although this only captures some forms of uncertainty. Over the last 14 days, the Leave estimate peaked at 53 around the 12th of June, and support has been moving towards Remain over the last week. We see less movement here than in snapshot polling releases, because we are able to pool together a larger number of observations and account for variation that is due to limited samples and other variation in who responds to surveys day to day. This pattern of stability with slow change is consistent with past research in the United States that has indicated that many apparent movements in polling go away once one accounts for instability in the kinds of voters responding to surveys.
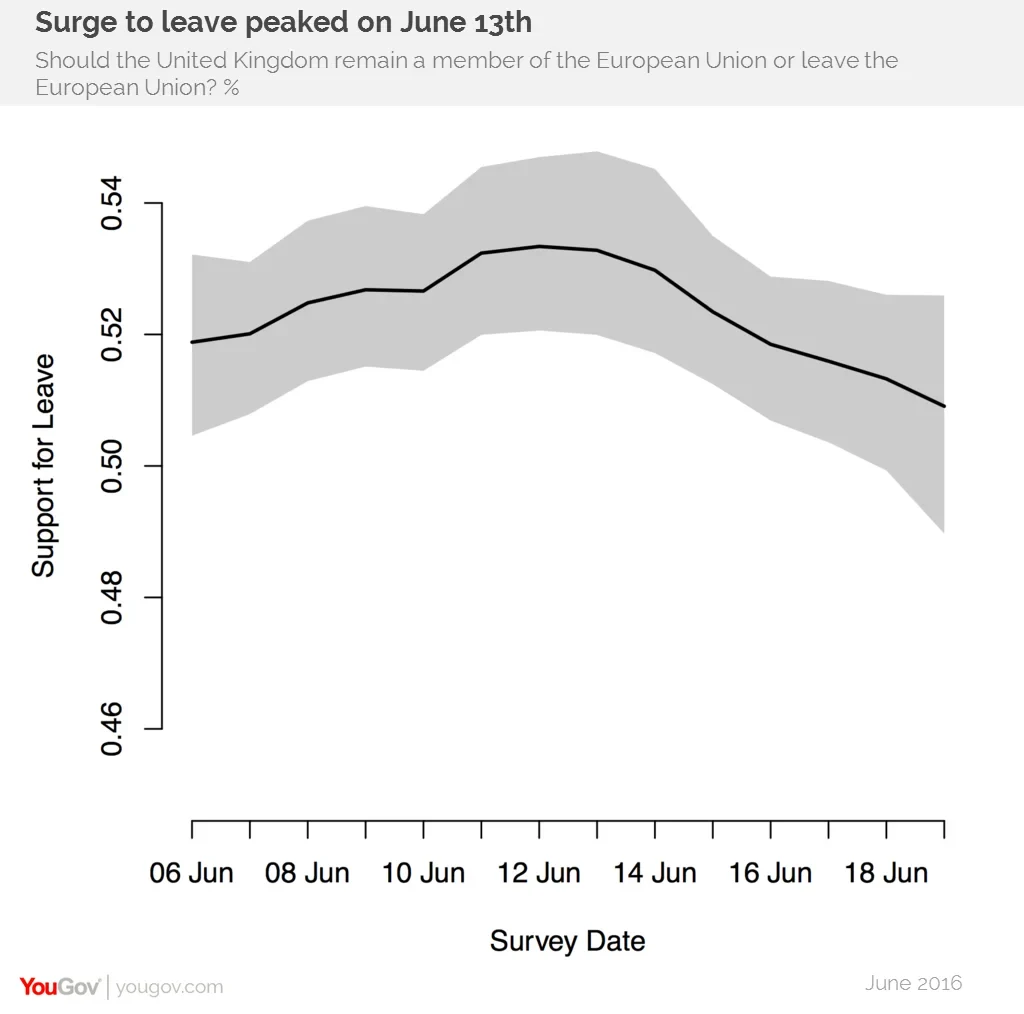
The method we are using allows us to break this result in a variety of ways. We have mapped support for Leave and Remain by parliamentary constituency. Support for Leave is strongest in the eastern coastal regions around The Wash, and the Humber and Thames Estuaries, but there is another more surprising area of strength in the areas surrounding Birmingham. Support for Remain is strongest in London, Scotland and other major towns and cities in England. The map visually understates the support for Remain because the areas supporting Remain tend to be dense urban areas, but the basic fact that Leave is in a strong position is rightly apparent from the map. We are not the first to note that there are strong relationships between vote intention, age, educational qualifications, and vote at the last general election, but we can say more about their interactions.
Unsurprisingly, UKIP voters nearly all support Leave, regardless of age and educational qualifications. Among supporters of the other parties older voters and those with lower levels of educational qualifications tend to support Leave at higher rates than younger voters and those with higher levels of qualifications, but the extent of these relationships vary. The associations between age, qualifications, and referendum vote intention are strongest among SNP/PC supporters, and generally weaker among Conservative and Liberal Democrat supporters, but for all parties except UKIP both qualifications and age are highly predictive of vote intention. These types of interactions make it particularly important for pollsters to make sure they are not just getting the right number of supporters of each party, but also the right number of supporters at different levels of qualifications and other variables.
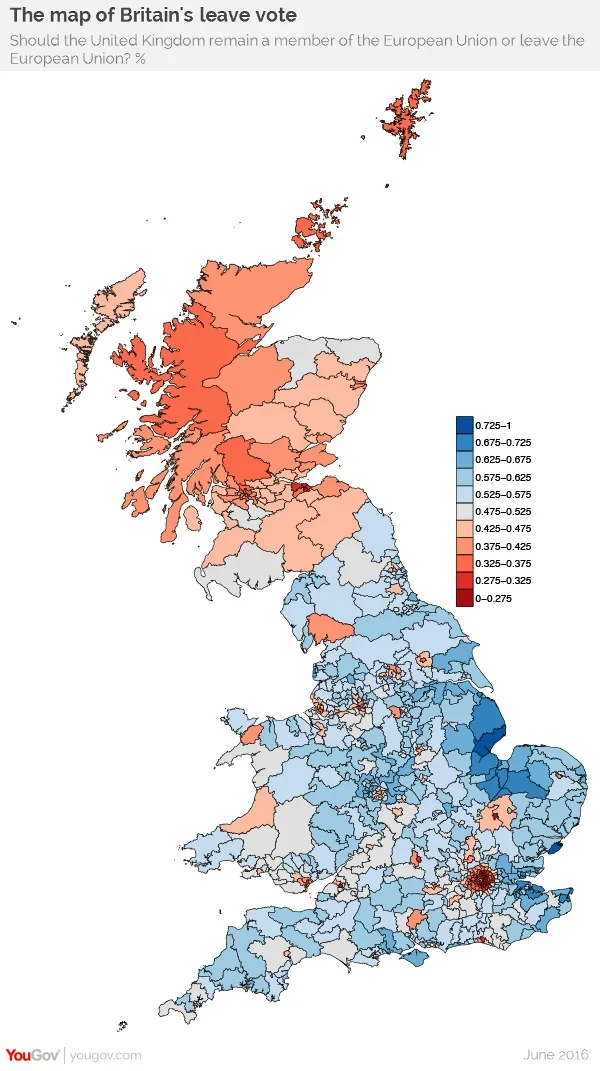
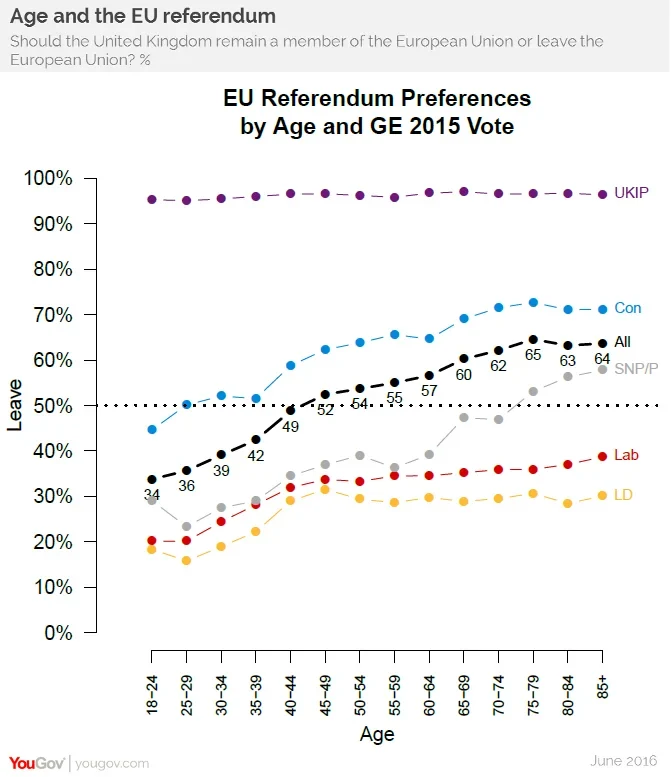
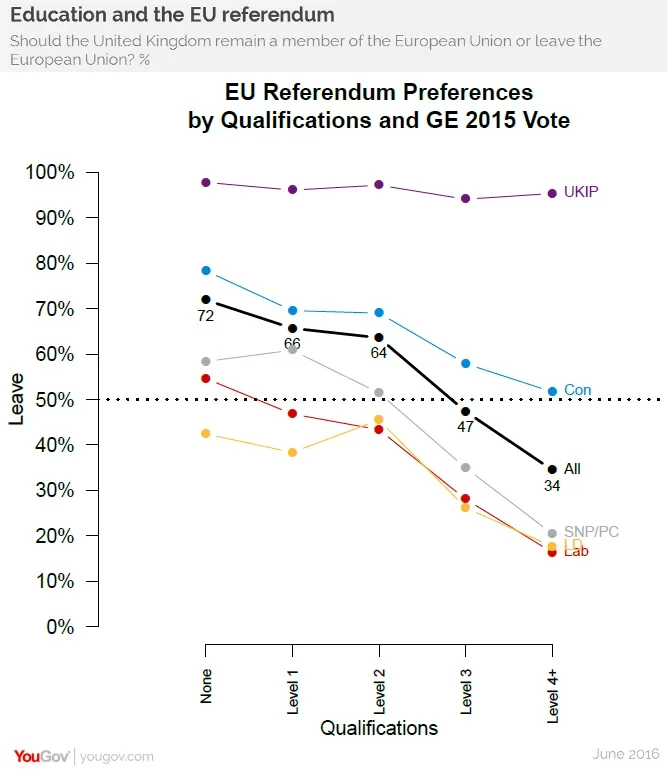
CAVEATS
One of us conducted YouGov’s inquiry into what went wrong with its 2015 general election polling and the other worked on the British Polling Council inquiry covering most major pollsters. Those inquiries had broadly shared conclusions that sample composition was the major source of problems with the general election polling. The fact that raw samples in both telephone and online polls are not representative of the entire population is well known, the problem is that the adjustments made to those raw samples to bring them inline with the population were inadequate. The turnout assumptions made by YouGov and other pollsters were also far off what happened, but
this was not directly responsible for the understatement of the Conservative versus Labour. We have addressed these issues in several ways. First, to address sample composition, we have used the multilevel regression and post-stratification approach describe above. This ensures that we are targeting not only the right number of individuals in each category of age, gender, qualifications, 2015 vote, and other variables, but also in all the voter types that are created by different combinations of those categories. Second, to address the problem of predicting patterns of turnout, we have used the BES face-to-face study to build a demographic model for turnout patterns in 2015, and have verified that those patterns also held in the 2010 election and the AV referendum, rather than using self-reported intention to turnout. Thus, if we have a problem, it will probably not be the same problem as last time. Still, there are a number of ways this estimation strategy might go awry, here are four broad categories of problems that might arise.
- Incorrect modelling assumptions. We may mis-estimate the relationships between demographic variables like age, gender and qualifications and 2015 turnout and vote choice. The BES (turnout) and YouGov data (vote choice) are not perfect, and we have to make some non-testable assumptions join these two sources of information about the population. We have tested a variety of models, which have yielded similar results.
- Old data. The 2011 Census data is five years out of date, and so the relationships between age, qualifications and other variables will have shifted to some degree. We have used the 2015 Annual Population Survey to update the distribution of some of our key variables to mitigate this problem. The 2015 election was one year ago, so some people have died and there is a new cohort of 18 year olds who could not vote at that election but can vote at this referendum. These discrepancies are likely to create only small errors.
- Turnout changes. The turnout model assumes that both the levels and the demographic patterns of turnout are similar to the 2015 general election. The turnout model also has to make an assumption about the extent to which it is the same people who turnout in 2015 and in this referendum. This could be a source of a large error if, for example, youth turnout increases substantially from its typically low level.
- Unrepresentative vote intention responses. If the voting behaviour of the individuals of a given type (e.g. female, age 31-25, etc) in the YouGov panel is unrepresentative of individuals of the same type in the broader UK population, that would cause problems for our estimates. As with any election, if respondents misreport their intentions or change their minds at the last minute, there is little we can do to fix that. The strategy we have followed yields a lot of information that we can use to diagnose what went right and what went wrong after the election. We have predictions for all of the groups of local authorities that the UK Census uses in the microdata sample, which means we will be able to easily see how well our estimates align with the geography of the result. After the election, we will report on how close this pre-election polling analysis came to the results, and what we can learn from this about how to proceed in future elections.